Researchers at Georgia Tech are advancing neural networks to imitate human decision-making by way of coaching them to show off variability and self assurance of their possible choices, very similar to how people perform, as demonstrated of their learn about revealed in Nature Human Behaviour. Their type, RTNet, now not most effective suits human efficiency in spotting noisy digits but in addition applies human-like characteristics akin to self assurance and proof accumulation, improving each accuracy and reliability. Credit score: SciTechDaily.comGeorgia Tech researchers have advanced a neural community, RTNet, that mimics human decision-making processes, together with self assurance and variability, making improvements to its reliability and accuracy in duties like digit reputation.People make just about 35,000 choices on a daily basis, starting from figuring out if it’s protected to go the street to selecting what to have for lunch. Each and every resolution comes to comparing choices, recalling an identical previous scenarios, and feeling fairly assured about the appropriate selection. What may seem to be a snap resolution in reality effects from amassing proof from the surroundings. Moreover, the similar particular person may make other choices in an identical situations at other occasions.Neural networks do the other, making the similar choices each and every time. Now, Georgia Tech researchers in Affiliate Professor Dobromir Rahnev’s lab are coaching them to make choices extra like people. This science of human decision-making is most effective simply being implemented to device finding out, however creating a neural community even nearer to the true human mind would possibly make it extra dependable, consistent with the researchers.In a paper in Nature Human Behaviour, a staff from the College of Psychology unearths a brand new neural community skilled to make choices very similar to people.Deciphering Choice“Neural networks decide with out telling you whether or not or now not they’re assured about their resolution,” stated Farshad Rafiei, who earned his Ph.D. in psychology at Georgia Tech. “This is without doubt one of the crucial variations from how folks make choices.”Massive language fashions (LLM), as an example, are susceptible to hallucinations. When an LLM is requested a query it doesn’t know the solution to, it is going to make up one thing with out acknowledging the artifice. In contrast, maximum people in the similar state of affairs will admit they don’t know the solution. Construction a extra human-like neural community can save you this duplicity and result in extra correct solutions.Making the ModelThe staff skilled their neural community on handwritten digits from a well-known pc science dataset referred to as MNIST and requested it to decipher each and every quantity. To decide the type’s accuracy, they ran it with the unique dataset after which added noise to the digits to make it more difficult for people to discern. To match the type efficiency towards people, they skilled their type (in addition to 3 different fashions: CNet, BLNet, and MSDNet) at the unique MNIST dataset with out noise, however examined them at the noisy model used within the experiments and in comparison effects from the 2 datasets.The researchers’ type trusted two key elements: a Bayesian neural community (BNN), which makes use of likelihood to make choices, and a proof accumulation procedure that assists in keeping observe of the proof for each and every selection. The BNN produces responses which can be rather other each and every time. Because it gathers extra proof, the buildup procedure can on occasion want one selection and on occasion any other. As soon as there may be sufficient proof to come to a decision, the RTNet stops the buildup procedure and comes to a decision.The researchers additionally timed the type’s decision-making pace to peer whether or not it follows a mental phenomenon referred to as the “speed-accuracy trade-off” that dictates that people are much less correct after they will have to make choices briefly.After they had the type’s effects, they in comparison them to people’ effects. Sixty Georgia Tech scholars seen the similar dataset and shared their self assurance of their choices, and the researchers discovered the accuracy fee, reaction time, and self assurance patterns have been an identical between the people and the neural community.“Normally talking, we don’t have sufficient human knowledge in current pc science literature, so we don’t understand how folks will behave when they’re uncovered to those photographs. This limitation hinders the advance of fashions that correctly mirror human decision-making,” Rafiei stated. “This paintings supplies probably the most greatest datasets of people responding to MNIST.”No longer most effective did the staff’s type outperform all rival deterministic fashions, nevertheless it additionally was once extra correct in higher-speed situations because of any other basic component of human psychology: RTNet behaves like people. For instance, folks really feel extra assured after they make right kind choices. With out even having to coach the type in particular to want self assurance, the type mechanically implemented it, Rafiei famous.“If we attempt to make our fashions nearer to the human mind, it is going to display within the conduct itself with out fine-tuning,” he stated.The analysis staff hopes to coach the neural community on extra numerous datasets to check its possible. In addition they be expecting to use this BNN type to different neural networks to permit them to rationalize extra like people. Ultimately, algorithms received’t simply be capable to emulate our decision-making talents, however may just even lend a hand offload one of the most cognitive burden of the ones 35,000 choices we make day-to-day.Reference: “The neural community RTNet reveals the signatures of human perceptual decision-making” by way of Farshad Rafiei, Medha Shekhar and Dobromir Rahnev, 12 July 2024, Nature Human Behaviour.
DOI: 10.1038/s41562-024-01914-8
AI Learns To Assume Like People: A Recreation-Changer in Device Finding out
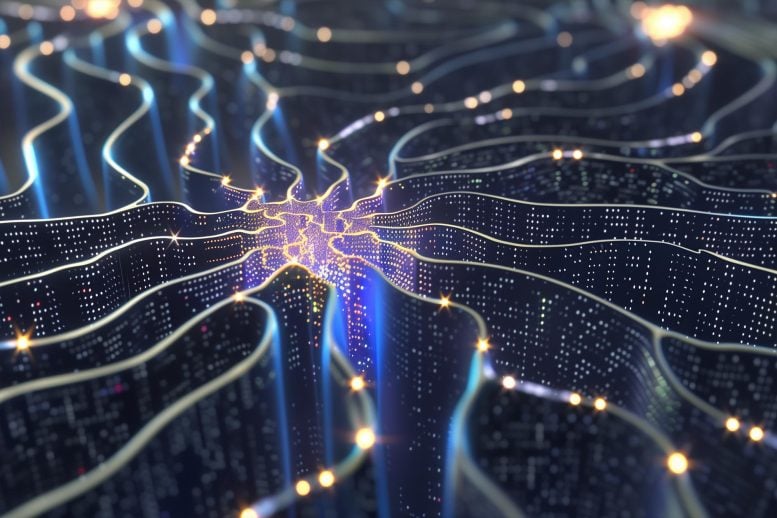