Abstract: Neural networks, irrespective of their complexity or coaching approach, practice a shockingly uniform trail from lack of know-how to experience in symbol classification duties. Researchers discovered that neural networks classify photographs via figuring out the similar low-dimensional options, corresponding to ears or eyes, debunking the belief that community finding out strategies are massively other.This discovering may pave the best way for growing extra effective AI coaching algorithms, doubtlessly lowering the numerous computational assets lately required. The analysis, grounded in news geometry, hints at a extra streamlined long run for AI construction, the place figuring out the average finding out trail of neural networks may result in less expensive and sooner coaching strategies.Key Information:Commonplace Studying Pathway: Neural networks, regardless of their design or measurement, discover ways to classify photographs via following a equivalent, low-dimensional trail.Attainable for Potency: The find out about’s insights counsel the potential of developing hyper-efficient AI coaching algorithms that require fewer computational assets.Foundation in Data Geometry: The use of news geometry allowed the researchers to match other networks on an equivalent footing, revealing their underlying similarities in finding out methods.Supply: College of PennsylvaniaPenn Engineers have exposed an surprising development in how neural networks — the techniques main as of late’s AI revolution — be informed, suggesting a solution to some of the necessary unanswered questions in AI: why those strategies paintings so neatly.Impressed via organic neurons, neural networks are pc techniques that absorb knowledge and educate themselves via time and again making small adjustments to the weights or parameters that govern their output, just like neurons adjusting their connections to each other. Finding an set of rules that may constantly in finding the trail had to educate a neural community to categorise photographs the usage of only a handful of inputs is an unresolved problem. Credit score: Neuroscience NewsThe ultimate result’s a style that permits the community to expect on knowledge it has no longer noticed prior to. Neural networks are getting used as of late in necessarily all fields of science and engineering, from drugs to cosmology, figuring out doubtlessly diseased cells and finding new galaxies.In a brand new paper printed within the Complaints of the Nationwide Academy of Sciences (PNAS), Pratik Chaudhari, Assistant Professor in Electric and Techniques Engineering (ESE) and core school on the Common Robotics, Automation, Sensing and Belief (GRASP) Lab, and co-author James Sethna, James Gilbert White Professor of Bodily Sciences at Cornell College, display that neural networks, regardless of their design, measurement or coaching recipe, practice the similar course from lack of know-how to reality when introduced with photographs to categorise. Jialin Mao, a doctoral pupil in Carried out Arithmetic and Computational Science on the College of Pennsylvania Faculty of Arts & Sciences, is the paper’s lead writer.“Assume the duty is to spot photos of cats and canine,” says Chaudhari.“You may use the whiskers to categorise them, whilst someone else would possibly use the form of the ears — you may presume that other networks would use the pixels within the photographs in several techniques, and a few networks for sure succeed in higher effects than others, however there’s a very sturdy commonality in how all of them be informed. That is what makes the end result so unexpected.”The outcome no longer handiest illuminates the internal workings of neural networks, however gestures towards the potential of growing hyper-efficient algorithms that might classify photographs in a fragment of the time, at a fragment of the price. Certainly, some of the best prices related to AI is the immense computational energy required to increase neural networks. “Those effects counsel that there might exist new techniques to coach them,” says Chaudhari. For example the possibility of this new approach, Chaudhari suggests imagining the networks as seeking to chart a path on a map.“Allow us to consider two issues,” he says.“Lack of know-how, the place the community does no longer know the rest about the right kind labels, and Fact, the place it will possibly as it should be classify all photographs. Coaching a community corresponds to charting a trail between Lack of know-how and Fact in likelihood house — in billions of dimensions. Nevertheless it seems that other networks take the similar trail, and this trail is extra like three-, four-, or five-dimensional.”In different phrases, regardless of the staggering complexity of neural networks, classifying photographs — some of the foundational duties for AI techniques — calls for just a small fraction of that complexity.“That is if truth be told proof that the main points of the community design, measurement or coaching recipes topic lower than we expect,” says Chaudhari.To reach at those insights, Chaudhari and Sethna borrowed gear from news geometry, a box that brings in combination geometry and statistics.By means of treating every community as a distribution of chances, the researchers had been ready to make a real apples-to-apples comparability a few of the networks, revealing their surprising, underlying similarities.“As a result of the peculiarities of high-dimensional areas, all issues are some distance clear of one every other,” says Chaudhari.“We advanced extra refined gear that give us a cleaner image of the networks’ variations.”The use of all kinds of ways, the group educated masses of hundreds of networks, of many alternative types, together with multi-layer perceptrons, convolutional and residual networks, and the transformers which might be on the middle of techniques like ChatGPT.“Then, this gorgeous image emerged,” says Chaudhari.“The output possibilities of those networks had been smartly clustered in combination on those skinny manifolds in gigantic areas.” In different phrases, the trails that represented the networks’ finding out aligned with one every other, appearing that they discovered to categorise photographs the similar means.Chaudhari gives two attainable explanations for this unexpected phenomenon: first, neural networks are by no means educated on random assortments of pixels.“Believe salt and pepper noise,” says Chaudhari. “This is obviously a picture, however no longer an overly fascinating one — photographs of exact gadgets like other folks and animals are a tiny, tiny subset of the gap of all imaginable photographs.”Put otherwise, asking a neural community to categorise photographs that topic to people is more straightforward than it sort of feels, as a result of there are lots of imaginable photographs the community by no means has to believe. 2d, the labels neural networks use are moderately particular. People crew gadgets into wide classes, like canine and cats, and shouldn’t have separate phrases for each and every specific member of each and every breed of animals.“If the networks had to make use of the entire pixels to make predictions,” says Chaudhari, “then the networks would have discovered many, many alternative techniques.” However the options that distinguish, say, cats and canine are themselves low-dimensional.“We consider those networks are discovering the similar related options,” provides Chaudhari, most probably via figuring out commonalities like ears, eyes, markings and so forth. Finding an set of rules that may constantly in finding the trail had to educate a neural community to categorise photographs the usage of only a handful of inputs is an unresolved problem.“That is the billion-dollar query,” says Chaudhari. “Are we able to educate neural networks affordably? This paper offers proof that we could possibly. We simply don’t know the way.”Investment: This find out about was once carried out on the College of Pennsylvania Faculty of Engineering and Carried out Science and Cornell College. It was once supported via grants from the Nationwide Science Basis, Nationwide Institutes of Well being, the Place of business of Naval Analysis, Eric and Wendy Schmidt AI in Science Postdoctoral Fellowship and cloud computing credit from Amazon Internet Services and products.Different co-authors come with Rahul Ramesh at Penn Engineering; Rubing Yang on the College of Pennsylvania Faculty of Arts & Sciences; Itay Griniasty and Han Kheng Teoh at Cornell College; and Mark Ok. Transtrum at Brigham Younger College.About this AI analysis newsAuthor: Ian Scheffler
Supply: College of Pennsylvania
Touch: Ian Scheffler – College of Pennsylvania
Symbol: The picture is credited to Neuroscience NewsOriginal Analysis: Closed get right of entry to.
“The learning strategy of many deep networks explores the similar low-dimensional manifold” via Pratik Chaudhari et al. PNASAbstractThe coaching strategy of many deep networks explores the similar low-dimensional manifoldWe increase information-geometric ways to research the trajectories of the predictions of deep networks right through coaching. By means of analyzing the underlying high-dimensional probabilistic fashions, we expose that the educational procedure explores an successfully low-dimensional manifold.Networks with a variety of architectures, sizes, educated the usage of other optimization strategies, regularization ways, knowledge augmentation ways, and weight initializations lie at the identical manifold within the prediction house.We find out about the main points of this manifold to seek out that networks with other architectures practice distinguishable trajectories, however different components have a minimum affect; better networks educate alongside a equivalent manifold as that of smaller networks, simply sooner; and networks initialized at very other portions of the prediction house converge to the answer alongside a equivalent manifold.
AI's Studying Trail: Sudden Uniformity Throughout Neural Networks – Neuroscience Information
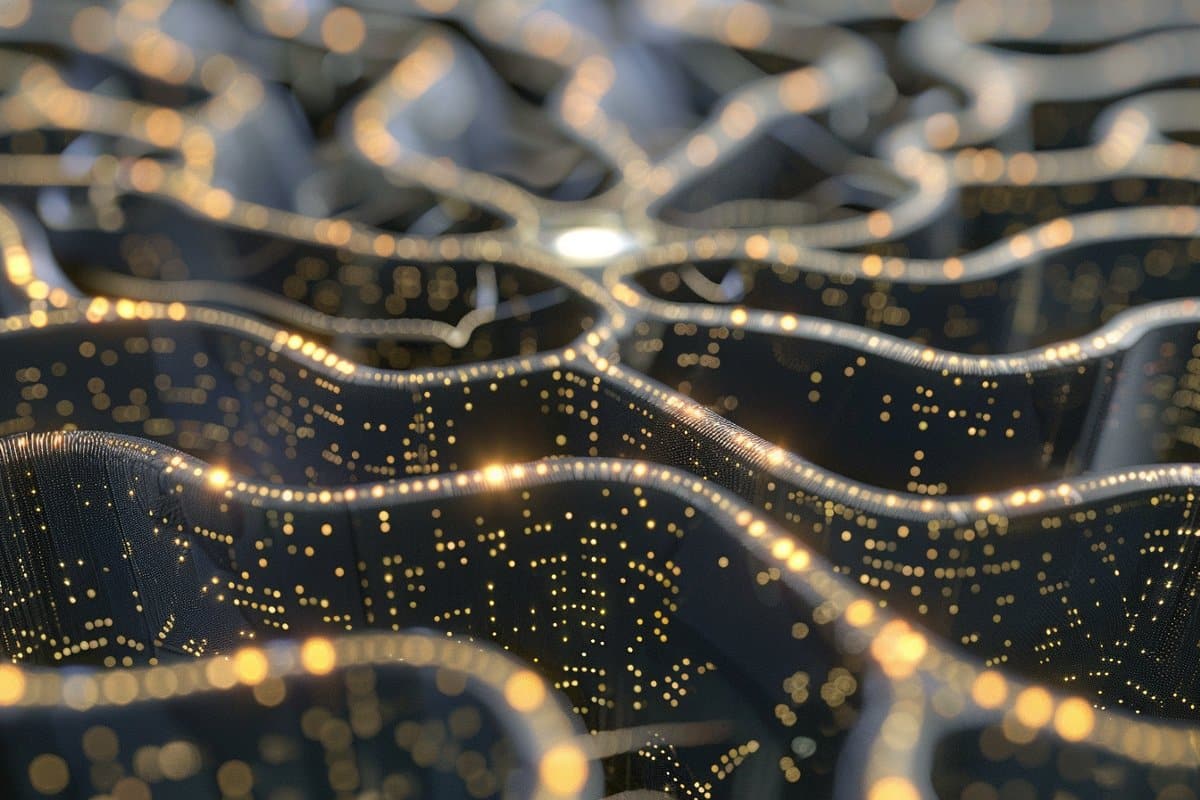