Researchers at MIT and the College of Washington have evolved a brand new strategy to make choices about brokers, bearing in mind constraints. This fashion, which is able to are expecting long run occasions from previous patterns, objectives to give a boost to the interplay between AI and human programs by way of figuring out and adapting to human idiosyncrasies and decision-making processes. Credit score: SciTechDaily.com A brand new approach can be utilized to are expecting the conduct of folks or AI that behaves out of regulate whilst operating on unknown duties. MIT and different researchers have evolved a gadget that displays the irrational or unacceptable conduct of an individual or an AI agent, in accordance with their computational difficulties. Their approach can assist are expecting what the agent will do one day, as an example, in a sport of chess. To construct AI programs that may engage neatly with folks, it is helping to have a excellent fashion of human conduct to start with. However folks have a tendency to behave irrationally when making choices. This irrationality, which may be very tough to fashion, is frequently because of computational difficulties. An individual does now not spend many years fascinated by one of the simplest ways to resolve a unmarried drawback. Making a New Means of Checking out Researchers at MIT and the College of Washington have evolved a option to fashion the conduct of an agent, both human or system, which calculates the unknown constraints that can impede the agent's skill to resolve issues. Their fashion can resolve an agent's boundaries by way of taking a look at just a small quantity in their previous efficiency. The outcome, referred to as a “predictive price range,” can be utilized to are expecting the agent's long run conduct. Practical Programs and Fashion Validation In a brand new paper, the researchers display how their approach can be utilized to resolve anyone's motion intentions from previous actions and are expecting what gamers will do one day. 'a sport of chess. Their strategies fit and even outperform different common decision-making strategies. In the end, the paintings may just assist scientists teach AI programs the way in which people do, which might assist those programs reply higher to their human opposite numbers. Having the ability to perceive what an individual does, after which base their intentions on that conduct, may just make an AI assistant extra helpful, says Athul Paul Jacob, a graduate pupil in electric engineering and pc science (EECS) and lead creator of the paper on. this fashion. “If we all know that an individual is set to make a screw up, after seeing how they’ve finished it sooner than, the AI assistant can step in and recommend a greater strategy to do it. Or the assistant can adapt to the weaknesses that the human companions have. Having the ability to imitate human conduct is the most important step in developing an AI assistant. who can in reality assist that particular person. in EECS and a member of the Pc Science and Synthetic Intelligence Laboratory (CSAIL). This find out about will likely be introduced on the World Convention on Modeling BehaviorResearchers were growing computational fashions of human conduct for a few years much less by way of including noise to the fashion. As a substitute of the agent all the time selecting the proper choice, the fashion could have the agent make the proper resolution 95 p.c of the time. To create their fashion, Jacob and his colleagues had been impressed by way of the early research of chess gamers. They discovered that gamers take much less time to assume sooner than appearing easy duties and that sturdy gamers have a tendency to spend extra time making plans than susceptible gamers in tough video games. the period of time an individual is considering the issue, is a superb proxy for a way folks reside,” says Jacob. They constructed a framework that may display the agent's intensity of pre-planning and use that data to fashion the agent's decision-making procedure. Step one of their approach comes to operating the set of rules for a suite period of time to resolve the issue being studied. As an example, if they’re learning a chess sport, they are able to let the chess sport run via a number of steps. On the finish, the researchers can see the choices made by way of the set of rules at each and every step. Their fashion compares those choices with the conduct of the agent to resolve the similar drawback. It is going to attach the choices of the agent with the choices of the set of rules and establish the step the place the agent stopped making plans. From right here, the fashion can resolve the price range of the agent, or how lengthy the agent will get ready for the issue. It could possibly use the price range procedure to turn how the agent can reply in fixing the similar drawback. An Interpretable SolutionThis approach will also be very helpful as a result of researchers can in finding all of the choices made by way of the issue fixing procedure with out doing further paintings. This procedure will also be carried out to any drawback that may be solved by way of a selected set of algorithms. “For me, essentially the most attention-grabbing factor was once that this explanatory price range may be very interpretive. They are saying that demanding situations require a large number of making plans or being a robust participant manner making plans for a very long time. Once we began doing this, we didn’t assume that our plan would have the ability to undertake the ones traits naturally,” Jacob says. predicting someone else's speech from their speech, and predicting the way forward for human-to-human chess video games. Their approach both matched or beat any other common approach in each and every take a look at. Additionally, the researchers discovered that their fashion of human conduct is in excellent settlement with the measures of the gamers' talent (in a chess fit) and the trouble of the duty. finding out (a trial-and-error approach extensively utilized in robotics). In the long run, they need to proceed to reinforce this paintings to succeed in without equal function of organising efficient AI brokers. Reference: “Modeling Boundedly Rational Brokers With Latent Inference Budgets” by way of Athul Paul Jacob, Abhishek Gupta and Jacob Andreas, ICLR 2024.
OpenReview This paintings was once supported, partly, by way of the MIT Schwarzman School of Computing Synthetic Intelligence for Augmentation and Productiveness program and the Nationwide Science Basis.
MIT’s New AI Fashion Predicts Human Habits With Uncanny Accuracy
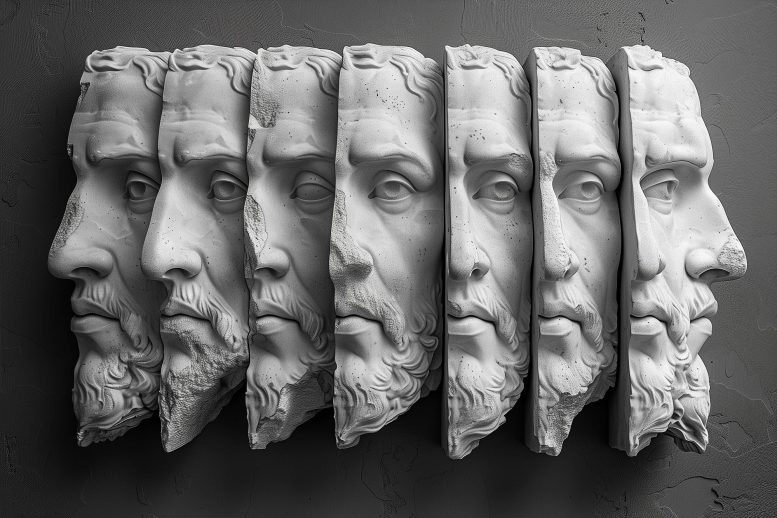